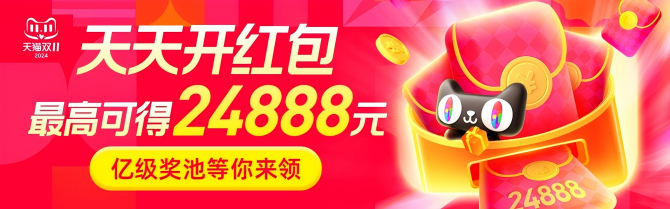
一、指导思想
# 只针对线性回归中的使用
算法的最优模型的功能:预测新的样本对应的值;
什么是最优的模型:能最大程度的拟合住数据集中的样本数据;
怎么才算最大程度的拟合:让数据集中的所有样本点,在特征空间中距离线性模型的距离的和最小;(以线性模型为例说明)
怎么得到最优模型:求出最优模型对应的参数;
怎么求解最优模型的参数:通过数学方法,得到目标函数(此函数计算数据集中的所有样本点,在特征空间中到该线性模型的距离,也就是损失函数),通过批量梯度下降法和随机梯度下降法对目标函数进行优化,得到目标函数最小值时对应的参数;
梯度下降法的目的:求解最优模型对应的参数;(并不是为了求目标函数的最小值,这一点有助于理解随机梯度下降法)
二、批量梯度下降法基础
1)批量梯度下降法的特点
运算量大:批量梯度下降法中的每一项计算:,要计算所有样本(共 m 个);
批量梯度下降法的梯度是损失函数减小最快的方向,也就是说,对应相同的 theta 变化量,损失函数在梯度方向上的变化量最大;
2)批量梯度下降法的思路
思路:计算损失函数的梯度,按梯度的方向,逐步减小损失函数的变量 theta,对应的损失函数也不断减小,直到损失函数的的变化量满足精度要求;
梯度计算:变形公式如下
梯度是优化的方向,损失函数的变量 theta 的变化量 = 学习率 X 当前梯度值
三、随机梯度下降法(Batch Gradient Descent)
1)基础理解
思路:随机抽取 n (一般 n = 总样本数 / 3)个样本,在每个样本的梯度方向上逐步优化(每随机抽取一个样本就对 theta 做一次递减优化)变量 theta;
分析:批量梯度下降法的优化,是整体数据集的梯度方向逐步循环递减变量 theta ,随机梯度下降法,是数据集中的一个随机的样本的梯度方向,优化变量 theta;
特点一:直接优化变量 theta,而不需要计算 theta 对应的目标函数值;
特点二:不是按整体数据集的梯度方向优化,而是按随机抽取的某个样本的梯度方向进行优化;
2)优化方向的公式
新的搜索方向计算公式(也即是优化的方向):;
此处称为搜索方向,而不是梯度的计算公式,因为此公式已经不是梯度公式,而表示优化损失函数的方向;
随机梯度下降法的搜索路径:
特点:
每一次搜索的方向,不能保证是损失函数减小的方向;
每一次搜索的方向,不能保证是损失函数减小最快的方向;
其优化方向具有不可预知性;
意义:
实验结论表明,即使随机梯度下降法的优化方向具有不可预知性,通过此方法依然可以差不多来到损失函数最小值的附近,虽然不像批量梯度下降法那样,一定可以来到损失函数最小值位置,但是,如果样本数量很大时,有时可以用一定的模型精度,换取优化模型所用的时间;
实现技巧:确定学习率(η:eta)的取值,很重要;
原因:在随机梯度下降法优化损失函数的过程中,如果 η 一直取固定值,可能会出现,已经优化到损失函数最小值位置了,但由于随机的过程不够好,η 又是各固定值,导致优化时慢慢的又跳出最小值位置;
方案:优化过程中让 η 逐渐递减(随着梯度下降法循环次数的增加,η 值越来越小);
3)η 的确定过程
一:如果 η = 1 / i_iters;(i_iters:当前循环次数)
问题:随着循环次数(i_iters)的增加,η 的变化率差别太大;
二:如果 η = 1 / i_iters + b);(b:为常量)
解决了 η 的变化率差异过大
再次变形:η = a / i_iters + b);(a、b:为常量)
分子改为 a ,增加 η 取值的灵活度;
a、b:为随机梯度下降法的超参数;
本次学习不对 a、b 调参,选用经验上比较适合的值:a = 5、b = 50;
学习率的特点
# 学习率随着循环次数的增加,逐渐递减;
# 这种逐渐递减的思想,是模拟在搜索领域的重要思路:模拟退火思想;
# 模拟退火思想:在退火过程中的冷却函数,温度与冷却时间的关系;
一般根据模拟退火思想,学习率还可以表示:η = t0 / i_iters + t1)
4)循环次数的确定
原则
将每个样本都随机抽取到;
将每个样本至少抽取 n 次,也就是总的循环次数一般为:lenX_b) * n;
具体操作
将变形后的数据集 X_b 的 index 做随机乱序处理,得到新的数据集 X_b_new ;
根据乱序后的 index 逐个抽取 X_b_new 中的样本,循环 n 遍;
四、实现随机梯度下降法
优化方向:,结果是一个列向量;
# array . dotm) == array . m
eta 取值:η = a / i_iters + b)
优化结束条件
批量梯度下降法:1)达到设定的循环次数;2)找到损失函数的最小值
随机梯度下降法:达到设定的循环次数
随机梯度下降法中不能使用精度来结束优化:因为随机梯度下降法的优化方向,不一定全都是损失函数减小的方向;
1)代码实现随机梯度下降法
模拟数据
import numpy as np import matplotlib.pyplot as plt m = 100000 x = np.random.normalsize=m) X = x.reshape-1, 1) y = 4. * x + 3. + np.random.normal0, 3, size=m)
批量梯度下降法
def Jtheta, X_b, y): try: return np.sumy - X_b.dottheta)) ** 2) / leny) except: return float'inf') def dJtheta, X_b, y): return X_b.T.dotX_b.dottheta) - y) * 2. / leny) def gradient_descentX_b, y, initial_theta, eta, n_iters=10**4, epsilon=10**-8): theta = initial_theta cur_iter = 0 while cur_iter < n_iters: gradient = dJtheta, X_b, y) last_theta = theta theta = theta - eta * gradient if absJtheta, X_b, y) - Jlast_theta, X_b, y)) < epsilon): break cur_iter += 1 return theta
# cur_iter:循环次数
# initial_theta:theta的初始化值
%%time X_b = np.hstack[np.oneslenX), 1)), X]) initial_theta = np.zerosX_b.shape[1]) eta = 0.01 theta = gradient_descentX_b, y, initial_theta, eta) # 输出:Wall time: 898 ms
theta # 输出:array[3.00280663, 3.9936598 ])
随机梯度下降法
1) 通过每一次随机抽取的样本,计算 theta 的优化方向def dJ_sgdtheta, X_b_i, y_i): return X_b_i.T.dotX_b_i.dottheta) - y_i) * 2
# X_b_i:是 X_b 中的一行数据,也就是一个随机样本,不在是全部的数据集
# y_i:对应的随机抽取的样本的真值
2) 随机优化过程 def sgdX_b, y, initial_theta, n_iters): # 计算学习率 eta t0 = 5 t1 = 50 # 定义求解学习率的函数 def learning_ratet): return t0 / t + t1) theta = initial_theta for cur_iter in rangen_iters): rand_i = np.random.randintlenX_b)) gradient = dJ_sgdtheta, X_b[rand_i], y[rand_i]) theta = theta - learning_ratecur_iter) * gradient return theta
# 此处的形参中不需要设置 eta 值了,eta 值随着循环的进行,在函数内部求取
# cur_iter:当前循环次数
# rand_i:从 [0, lenX_b)) 中随机抽取的一个数
# gradient:一次循环中,随机样本的优化方向
# learning_ratecur_iter) * gradient:一次循环的 theta 的变化量
3)给初始化数值,预测数据 %%time X_b = np.hstack[np.oneslenX), 1)), X]) initial_theta = np.zerosX_b.shape[1]) theta = sgdX_b, y, initial_theta, n_iters=lenX_b)//3) # 输出:Wall time: 287 ms
4)查看最终优化结果 theta # 输出:array[2.9648937 , 3.94467405])
2)封装与调用自己的代码
封装:已规范循环次数(代码中的红色字样)
1 def fit_sgdself, X_train, y_train, n_iters=5, t0=5, t1=50): 2 """根据训练数据集X_train, y_train, 使用梯度下降法训练Linear Regression模型""" 3 assert X_train.shape[0] == y_train.shape[0], 4 "the size of X_train must be equal to the size of y_train" 5 assert n_iters >= 1 6 7 def dJ_sgdtheta, X_b_i, y_i): 8 return X_b_i * X_b_i.dottheta) - y_i) * 2. 9 10 def sgdX_b, y, initial_theta, n_iters, t0=5, t1=50): 11 12 def learning_ratet): 13 return t0 / t + t1) 14 15 theta = initial_theta 16 m = lenX_b) 17 18 for cur_iter in rangen_iters): 19 indexes = np.random.permutationm) 20 X_b_new = X_b[indexes] 21 y_new = y[indexes] 22 for i in rangem): 23 gradient = dJ_sgdtheta, X_b_new[i], y_new[i]) 24 theta = theta - learning_ratecur_iter * m + i) * gradient 25 26 return theta 27 28 X_b = np.hstack[np.oneslenX_train), 1)), X_train]) 29 initial_theta = np.random.randnX_b.shape[1]) 30 self._theta = sgdX_b, y_train, initial_theta, n_iters, t0, t1) 31 32 self.intercept_ = self._theta[0] 33 self.coef_ = self._theta[1:] 34 35 return self
# n_iters:对所有数据集循环的遍数;
调用自己封装的代码
获取原始数据
import numpy as np import matplotlib.pyplot as plt from sklearn import datasets boston = datasets.load_boston) X = boston.data y = boston.target X = X[y < 50.0] y = y[y < 50.0]
数据分割
from ALG.data_split import train_test_split X_train, X_test, y_train, y_test = train_test_splitX, y, seed=666)
数据归一化
from sklearn.preprocessing import StandardScaler standardScaler = StandardScaler) standardScaler.fitX_train) X_train_standard = standardScaler.transformX_train) X_test_standard = standardScaler.transformX_test)
# 数据归一化,主要是将训练数据集(X_train)和测试数据集(X_test)归一化;
使用线性回归算法:LinearRegression
from LR.LinearRegression import LinearRegression lin_reg = LinearRegression) %time lin_reg.fit_sgdX_train_standard, y_train, n_iters=2) lin_reg.scoreX_test_standard, y_test) # 输出:Wall time: 10 ms 0.7865171620468298
# 问题:通过score)函数得到的 R^2 值,也就是准确度过小
# 原因:对所有的 X_train_standard 循环优化的遍数太少:n_iters=2
循环遍数改为 50:n_iters=50
%time lin_reg.fit_sgdX_train_standard, y_train, n_iters=50) lin_reg.scoreX_test_standard, y_test) # 输出:Wall time: 143 ms 0.8085728716573835
循环遍数改为 100:n_iters = 100
%time lin_reg.fit_sgdX_train_standard, y_train, n_iters=100) lin_reg.scoreX_test_standard, y_test) # 输出:Wall time: 502 ms 0.8125954368325295
总结:随着循环遍数的增加,模型的准确度也随着增加;
3)调用 scikit-learn 中的算法模型
SGDRegressor:该算法虽是名为随机梯度下降法的回归器,但其只能解决线性模型,因为,其被封装在了 linear_model 线性回归模块中;
学习scikit-learn中的算法的过程:掉包 – 构建实例对象 – 拟合 – 验证训练模型的效果(查看准确度)
实现过程:前期的数据处理与步骤(2)相同
from sklearn.linear_model import SGDRegressor sgd_reg = SGDRegressor) %time sgd_reg.fitX_train_standard, y_train) sgd_reg.scoreX_test_standard, y_test) # 输出:Wall time: 16 ms 0.8065416815240762
# 准确度为0.8065 左右,此时循环遍数使用默认的 5 遍:max_iter = 5
修改循环遍数:max_iter = 100
sgd_reg = SGDRegressormax_iter=100) %time sgd_reg.fitX_train_standard, y_train) sgd_reg.scoreX_test_standard, y_test) # 输出:Wall time: 8 ms 0.813372455938393
4)总结
与自己写的算法相比,scikit-learn中的算法的实现过程更加复杂,性能更优:
通过计算时间就可以看出:n_iters=100时,自己的算法需要 502ms,scikit-learn中的算法需要 8ms;
自己所学的封装好的算法,只是为了帮助理解算法的原来,而scikit-learn中使用了很多优化的方案